In the realm of biotechnology, the ability to design antibodies tailored to target specific proteins or pathogens has been a long-standing challenge. Antibodies, the powerful workhorses of our immune system, have proven invaluable in developing targeted therapies and diagnostic tools. However, the traditional process of antibody production is time-consuming, labor-intensive, and often yields suboptimal results. Enter the world of AI-driven protein design, a technological breakthrough that could revolutionize the way we create antibodies.
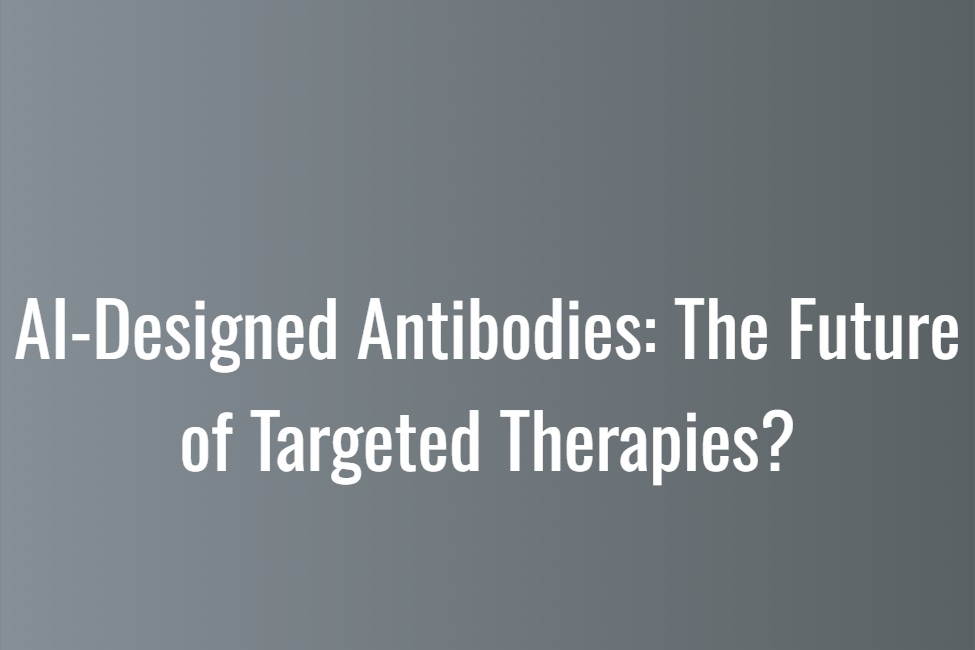
The Antibody Conundrum
Traditionally, antibody production has relied on immunizing animals, such as mice or rabbits, with the target protein or pathogen. This process triggers an immune response, leading to the production of antibodies by animal’s immune cells. These antibodies are then harvested and purified for further use. However, this approach is not without its limitations. It can be costly, time-consuming, and may not always yield antibodies with the desired specificity or affinity for the target.
AI-Designed Antibodies to the Rescue
Recent advancements in artificial intelligence (AI) and protein structure prediction have paved the way for a more efficient and precise approach to antibody design. Researchers have successfully adapted diffusion models, a type of AI system originally developed for generating images, to design antibodies against specific targets.
The process involves training the AI model on a vast dataset of known antibody structures and their corresponding amino acid sequences. By learning the intricate relationships between sequence and structure, the model can then generate new antibody sequences tailored to bind and potentially neutralize a specific target protein or pathogen.
Advantages of AI-Designed Antibodies
- Precision and Specificity: AI-designed antibodies can be engineered to have high specificity and affinity for their intended targets, minimizing off-target effects and improving therapeutic efficacy.
- Time and Cost Efficiency: Bypassing the animal immunization process significantly reduces the time and resources required for antibody development, accelerating the timeline from discovery to clinical application.
- Versatility: AI models can be trained to design antibodies against virtually any target, from viral proteins to cancer-specific antigens, expanding the potential applications in both therapeutic and diagnostic settings.
- Optimization: AI algorithms can explore a vast sequence space, allowing for the optimization of antibody properties, such as stability, solubility, and manufacturability, improving their therapeutic potential.
Challenges and Future Directions
While AI-driven antibody design holds immense promise, several challenges remain. Ensuring the accuracy and reliability of the AI models is crucial, as errors in protein structure prediction could lead to ineffective or potentially harmful antibody designs. Additionally, extensive experimental validation and clinical trials will be necessary to establish the safety and efficacy of AI-designed antibodies.
As the field continues to evolve, researchers are exploring ways to integrate AI approaches with other cutting-edge technologies, such as protein engineering and directed evolution, to further refine and optimize antibody designs. The synergy between AI and experimental techniques could pave the way for a new era of personalized and targeted therapies, revolutionizing the way we combat diseases and address unmet medical needs.
AntibodyDesign, #AI, #ProteinEngineering, #TargetedTherapies, #Biotechnology, #Immunology, #DrugDiscovery, #PrecisionMedicine, #StructuralBiology, #FutofHealthcare
Discover more from TechResider Submit AI Tool
Subscribe to get the latest posts sent to your email.