Most emerging technologies like Machine learning (ML) and Artificial Intelligence (AI) are going hand in hand to bring the revolution in the healthcare industry.
ML is the sub-category of Artificial Intelligence (AI) that focuses on improving the performance of the medical team constructively with less time and more speed using large-scale medical data.
Undeniably, the Healthcare industry has always been open to adopting new technologies. From Big data tools and data analytics to EMR/EHR, the healthcare industry has always been a strong supporter of innovative new technological advancements.
The ML and AI technologies are efficient in handling complex and large amounts of data. The automated ML system provides high-quality healthcare services and facilities to the patients.
So, let’s explore machine learning’s benefits.
What are the benefits of Machine learning in healthcare?
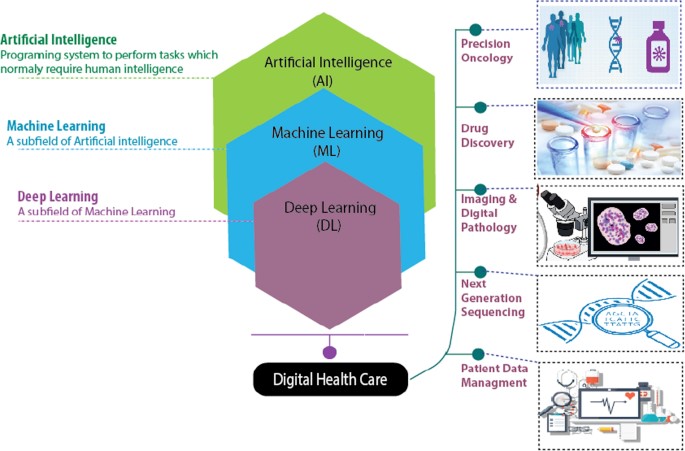
The use of machine learning in healthcare has proved very beneficial.
- Large amounts of data handling, processing, report generating, diagnosis and health solution options are all important tasks that are effectively handled by the ML system with much ease and security.
- It enables healthcare professionals to save time so that they can focus on the other key activities related to patient care solutions.
- The data and the algorithm makes various patterns which are detected by the computer through ML in order to predict care outcomes and adequate diagnosis strategy
- ML performs the same tasks more efficiently than the manual way which saves ample time for the medical professionals
- The ML trains computers to interpret the data and provide the solutions automatically
- Various conditions and points allow ML to interpret data in the administered or unadministered conditions
- Machine Learning Helps in patients’ involvement in the process like the physical presence of the patient during the treatment
- Regular health concerned tips or messages are sent to the patient to monitor the health conditions which saves time for the patients
What are the top machine learning use cases in healthcare?
Therapeutic Imaging
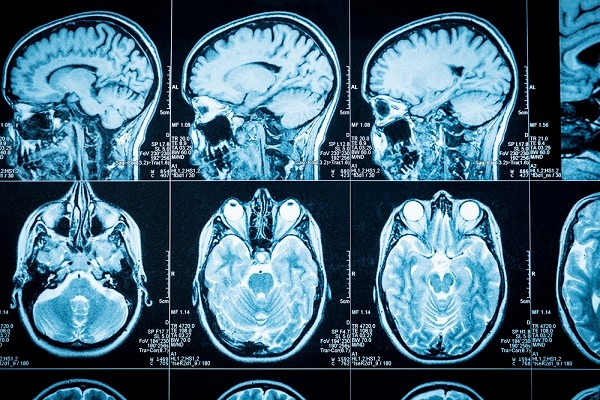
Magnetic Resonance (MR) and Computed Tomography (CT) are the processes used in therapeutic image recognition. It is the process for image analysis, disease detection and disease prediction.
The clear specification is made by the study of a combination of image data such as calculation of the tissue size, volume and shape.
The algorithm is structured to make early detection of the diabetic possibilities, early stage of Alzheimer and ultrasound detection of the breast clots. Furthermore, the radiology and pathology areas are also detected seamlessly.
The convolutional neural networks (CNNs) allow the medical experts to simplify the complex data analysis and rectify the health issues of the patients with immediate effect and with conventional accuracy.
The reports have proved that CNN’s detection of Atopic dermatitis (eczema) is the disease in dermatology images with more than 15% accuracy than the physical examination by the experts.
Malignant rate
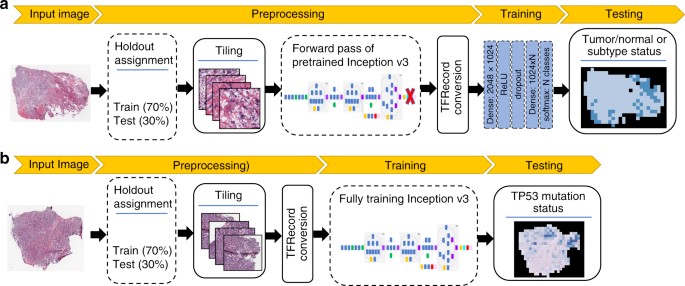
The development of the tumour can be detected. The size of the tumour can be tracked and the phases of tumour development which can go unnoticed can be reported accurately by the increase in the data given by the algorithm with more CT and MRI scans.
The algorithm gives such accurate readings that there is no possibility of missing out on any tumorous cell, every detail information like how fast and in what range the tumour is growing in the body, all such data is accurately captured.
Oncology and skin specialists demand the use of algorithm detection of chronic diseases as they support and guide medical experts with early detection of the disease. Many lives can thus be saved with the machine learning technology, suggests the experts.
Data Analytics in the healthcare
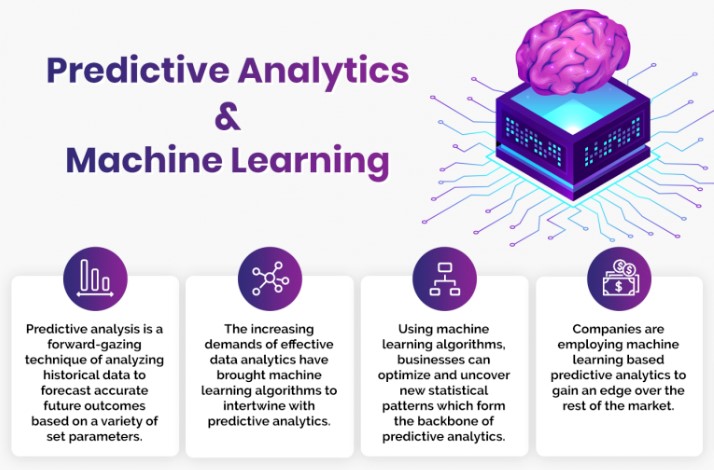
Machine learning can analyze the Electronic health records (EMR) that contain huge structured and unstructured data, clinical reports, medication tracks, diagnoses and lab reports with phenomenal speed and accuracy.
Wearable devices and also smartphones are supported by mobile application programs allowing the user to track their health records and get accurate readings and reports.
The device can successfully measure the pulse rate, body temperature, respiration, mobility of the patient, heart rate, Blood pressure reading etc.
Patient Churn Analysis
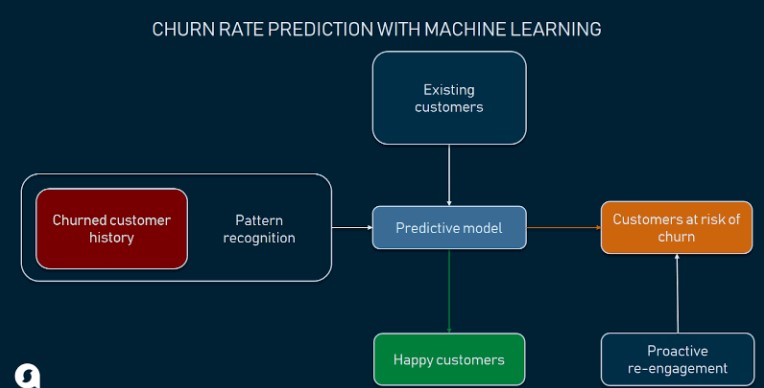
The churn analysis is the matrix that keeps track of the patients’ activities and the number of visits to any particular hospital. The data collected from the source about the patient’s visit to the doctor gives accurate feedback.
The prediction is done by the analysis report on whether or not the patient will revisit the same doctor in future. The possibilities of the patients who won’t visit the same doctor again are studied and measures are taken to develop opportunities that can pull back the patients for future visits.
Research and Development
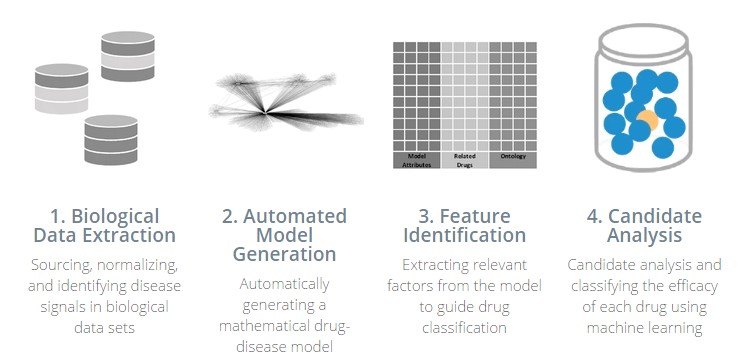
The new technology is advancing the discovery and enhancement of drugs.
The genomic, clinical and population data is processed to detect the viable drug by the algorithms of the system. The large data is studied in detail by the pharmaceutical companies for their future business growth.
Introduce new anomalies
Machine learning trains the computer to identify the patterns with the help of the data and the algorithm to understand the data. This process of learning from the data is called training and the result we achieve is the model. The models used in this technique are classified.
The main objective of this classification is to tag the data. The clustered data is the undefined or unlabelled data where we don’t have any outcome from the data which is widely in the collection.
With the study of distinctive patterns, the regressive data is collected in order to check whether the patient with chronic disease will come back again for the treatment or not.
While obtaining all the labelled or unlabeled data information, we come across anomalies. Through the statistical techniques, all the patterns of the data are analyzed and the unrequired data bites are separated which identifies the fraud activities in the various clinical operations.
All unstructured and unlabelled data is removed so it doesn’t affect the model in any way. Several statistical techniques help to tackle the new anomalies.
Conclusion
Autonomous robotic surgeries and personalized medical assistance with ML have supported greatly in fighting against diseases and getting back to life with a much higher spirit and energy.
The confidence is gained back by the patient, and technology has widely changed the scene on chronic diseases as well. With proper diagnosis and treatment, the deadliest disease can be cured with the right treatment.
Robots are performing surgeries on humans with a high success rate. Soon we can also imagine having the healthcare software development companies introducing applications to our phones which can identify the symptoms and guide us for future treatment.
Discover more from TechResider Submit AI Tool
Subscribe to get the latest posts sent to your email.